"Recursive ensemble feature selection provides a robust mRNA expression signature for myalgic encephalomyelitis/chronic fatigue syndrome" (Metselaar, Yim, Abarkan, Henneman, Velde, Schönhuth, Bosch, Kraneveld, & Lopez-Rincon, 2021)
https://www.nature.com/articles/s41598-021-83660-9
The 23 predictor genes that emerged were:
KCNA2, ARRB1, PRG4, ABCE1, DENND5A, ECT2, MAPK4, GOLGA4, CCR4, CORO6, IL2RB, NMNAT1, ADAM22, PHKA2, PTPRM, HLA-DQA1, OGG1, SPAST, BRAT1, STRBP, COL3A1, NCOA6, and UTP4.
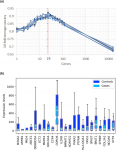
https://www.nature.com/articles/s41598-021-83660-9
Discussion
ME/CFS is a chronic disorder characterized by persistent, disabling fatigue for which no diagnostic or prognostic test nor complete treatment is available. Several studies have sought to define biomarkers for ME/CFS by performing differential mRNA expression or DNA methylation analysis. However, as Byrnes et al.15 pointed out, these results were study-dependent and no definitive biomarkers were found. We used a state-of-the-art machine learning technique to distinguish ME/CFS patients from healthy controls across different platforms, several cohorts and on different levels of gene expression regulation. To our knowledge, this was the first time such a technique was used in mRNA expression data and validated in DNA methylation data.
In this study, we implemented the REFS algorithm on public mRNA expression data and found 23 genes whose changes in expression levels were able to distinguish ME/CFS patients from healthy controls. The 23 predictor genes differentiated between cases and controls with 91.57% global accuracy and returned a ROC AUC of 0.92. In addition, 48 CpG methylation sites associated with these genes were predictive of ME/CFS in four merged DNA methylation studies. Moreover, all 23 candidate genes were downregulated in ME/CFS patients while DNA methylation of almost all 48 CpG sites was enhanced. This inverse correlation between mRNA expression and DNA methylation, across different samples and studies, legitimizes the results of our study. As previously demonstrated25, REFS identifies a more accurate, robust gene signature than previous methods. Comparing the gene signature returned by three different methods, based on the same data, REFS outperformed both IWGCNA and univariate analysis in separating ME/CFS patients and healthy controls with a ROC AUC of 0.92. The AUC of the gene signature applied to a different platform was 0.95, and the AUC even reached 0.97 when plotting the sensitivity and specificity of the 48 predictor CpG sites.
To show the relevance of the returned predictor genes, we investigated the biological functions of ten encoded proteins active in immune pathways. This decision was based on the mRNA expression being measured in PBMCs and the literature pointing towards an important role for the immune system in ME/CFS. Sotzny et al.42 reviewed autoimmunity in ME/CFS, concluding that immunologic and metabolic alterations were often reported. The authors stress the potential importance of autoantibodies in the disorder and the proposed role of preceding infections. Downregulation of ABCE1, one of the encoded proteins identified in our study, concurs with the presence of previous viral infections, as the protein inhibits RNase L’s viral RNA degrading activity. Similarly, ARRB1 protein was decreased after Epstein-Barr virus-infection in mice43. Its downregulation in our study concurs with this finding. Recently, Mandarano et al.44 described evidence of immune involvement in their study of 53 ME/CFS patients. They specifically focused on T cells, showing that CD8+ T cells derived from patients had lower mitochondrial membrane potential, which points towards T cell exhaustion. PHKA2 is necessary for the first step in breaking down glycogen to glucose. Its downregulation could contribute to impaired glycolysis in immune cells. In ME/CFS, CD4+ and CD8+ T cells had impaired resting glycolysis, and plasma glucose was reduced. CD8+ T cells showed an impaired metabolic response to activation44. Another study found that glycogen metabolism regulates the immune functions of dendritic cells45. Inhibiting glycogen phosphorylase impaired their ability to produce inflammatory cytokines and stimulate T cells. These findings combined suggest that reduced PHKA2 in ME/CFS might inhibit glycogen phosphorylase activation and thus dendritic cell functioning.
Several (subunits of) immune cell receptors were also part of our gene signature and downregulated in ME/CFS. IL2RB, CCR4 and HLA-DQA1 are vital elements in proper immune response, dysregulation, whether up or down, could be evidence of a disturbed immune system or be the cause of it. The same holds true for decreased MAPK4 expression, an ubiquitous transducer of intracellular signals in response to immune cell receptor binding. Further downstream, GOLGA4 was upregulated in response to macrophage LPS activation to increase TNF secretion. TNF is the main pro-inflammatory cytokine secreted by inflammatory macrophages, and its release is important for enhancing the activation and recruitment of T cells, ensuring robust innate and adaptive immune responses. In our study however, GOLGA4 was downregulated in PBMCs of ME/CFS patients, potentially causing impaired TNF secretion and subsequently an impaired immune response to inflammation. Furthermore, decreased expression of PRG4 leads to reduced anti-inflammatory action of this protein. By binding immune cells receptors, PRG4 prevents activation by low levels of circulating pro-inflammatory cytokines. PRG4 could thus be important in low-grade inflammation causing ME/CFS46.
Finally, evidence has emerged that oxidative stress levels are raised in ME/CFS, for example in response to exercise, perhaps causing some of the symptoms seen in ME/CFS47,48. DNA damage caused by exposure to ROS leads to mutagenesis or cell death, OGG1 specifically repairs this DNA damage. OGG1 depletion in human monocyte-derived dendritic cells inhibited enhanced cell surface molecule-expression and secretion of pro-inflammatory cytokines upon exposure to 8-oxoguanine base lesions. This suggests that OGG1 is important for dendritic cell activation in response to ROS49. Concurrently, 8-oxoguanine base lesions did not cause acute or systemic inflammation in Ogg1-deficient mice50. As we found that OGG1 is downregulated in ME/CFS patients, while oxidative stress levels are increased, DNA damage might be increased, which in turn causes the release of danger associated molecular patterns (DAMPs) and activates the innate immune system. We can conclude, from the various roles of these ten genes, that their downregulation may not only contribute to immune activation, but also towards a general dysregulation of the immune response. Whether all genes are causative of the ME/CFS phenotype, or some are mere consequences of immune mayhem in ME/CFS patients remains to be investigated.
Our results return a promising gene signature for ME/CFS that needs to be validated in a well characterized clinical cohort to study its use as a diagnostic tool. In this cohort, the number of cases and controls should be balanced, as the current study suffers from cases outnumbering controls in both the mRNA expression and DNA methylation datasets. We compensated for the imbalance with stratified folds in the cross-validation. Finally, the investigation of the predictor genes has thus far been limited to a literature review. In vitro experiments with PBMCs should provide additional information regarding gene function.
To conclude, we found a mRNA expression signature of 23 genes for ME/CFS capable of separating cases and controls. These candidate genes could potentially be used as biomarkers for diagnostic purposes. In addition, ten of these genes could be interpreted in the context of a derailed immune system in ME/CFS. Those genes could be investigated further for target finding and development of future treatments for ME/CFS.
The 23 predictor genes that emerged were:
KCNA2, ARRB1, PRG4, ABCE1, DENND5A, ECT2, MAPK4, GOLGA4, CCR4, CORO6, IL2RB, NMNAT1, ADAM22, PHKA2, PTPRM, HLA-DQA1, OGG1, SPAST, BRAT1, STRBP, COL3A1, NCOA6, and UTP4.
Results of the REFS algorithm run ten times on the mRNA expression data of 118 samples from the CAMDA dataset. (a) The optimal number of predictor genes to distinguish 93 cases from 25 controls was 23 (red vertical line). (b) mRNA expression levels of the 23 predictor genes for 93 cases (light blue) and 25 controls (dark blue) in box-and-whisker plots. Outliers were omitted for visualization purposes.
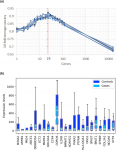
Last edited: